As covered in our previous article, new technologies and new ways of thinking about data and its representation have profoundly changed the architecture of Information Systems..
Data integration solutions have gone from the man-orchestra to the conductor model.But to play which score?
That of a well-tuned quartet? Or that of a chamber orchestra, playing a limited selection of instruments?
On the contrary, the ELT vision, also called "delegation of transformations", is once again an agile and relevant alternative for dealing with the diversity of data and architectures.
It makes possible a more sonorous and richer work, a cleverly orchestrated symphony.
A powerful, efficient symphony, capable of coordinating an unlimited number of instruments over time.
This is what we will discuss in this article: ELT, a real response to the challenges of performance and industrialization of flows.
A need for increased performance
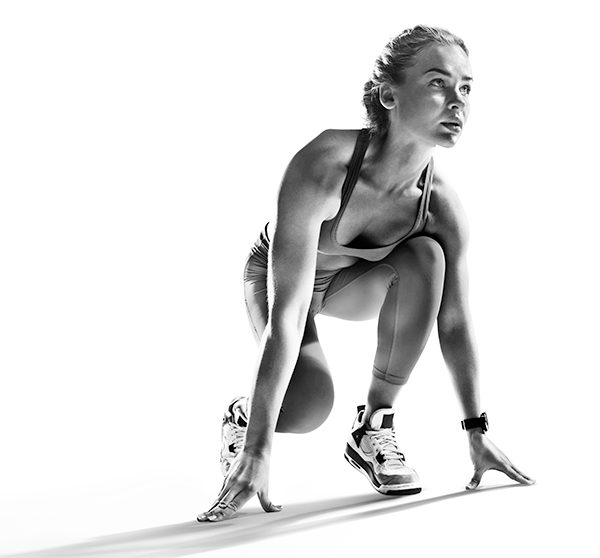
This isn’t anything new, performance is a more meaningful requirement than it was at the genesis of the ETL.
While in the past it was necessary to keep data extractions in a production window of a few hours at a weekly rate, even daily for the most ambitious among us, it is now unthinkable for some organizations to have a deadline of more than a few seconds, if not a few milliseconds between two data extractions!
The world is changing, and what was once a luxury is now regarded as a basic commodity.
Any resemblance to any other area of daily life would be completely fortuitous, though…
At the same time, it is useful to recall the phenomenal explosion in the volume of data available to organizations.
According to IDC, whose data is formatted by Statica (article Journal Du Net)
"The global volume of data will be multiplied by a further 3.7 between 2020 and 2025, then by 3.5 every five years until 2035, to reach the dizzying sum of 2,142 zettabytes"
The rise of Big Data is only the beginning of this revolution in technology and data volumes.
What this new technological wave has shown us is that computing power that was previously reserved for scientific research or for projects classified as "top secret" is now within the reach of the smallest of organizations.
Traditional ETL can seem poor in front of a Spark cluster or use of Google Big Query.
Performance is at the very heart of the Big Data revolution.
In this context, only an intelligent use of the native functionalities of each platform can provide satisfaction in terms of performance.
Delegation of transformation (ELT) is therefore, as we explained, not only an alternative, but a necessity.
No traditional ETL today claims to replace a Spark cluster or perform the transformation in place of the target database, be it Google BigQuery or any other technology.
Performance must be secure and immediate, and as close as possible to the data.
It is no longer possible to move the data into an engine, because the data analyzes are done on terabytes, even petabytes of data, and sometimes on raw, non-aggregated, or even unstructured data, which reinforces the required velocity in processing.
Essential control of cloud costs
Now that that’s settled, we have to add a little caveat to this symphony: performance, yes, but not at any price.
Gone are the days when the budgetary floodgates were wide open, when investment was the main objective of CIOs and the only limit was that of Moore's Law!
We once believed that Big Data would be a way out, a useful pretext for ever more pharaonic investments. But the budgetary reality has unfortunately calmed the ardor of the most tech-hungry.
The Cloud once seemed like a great idea to control costs, or simply move them from one budget line to another. There is debate.
However, even the soft, light sound of the Cloud Magic Flute does not alleviate the need to master the sheet music. On the contrary, the simplicity of the model, where all you need is a slider to adjust the power, can quickly lead to budgetary slippages.
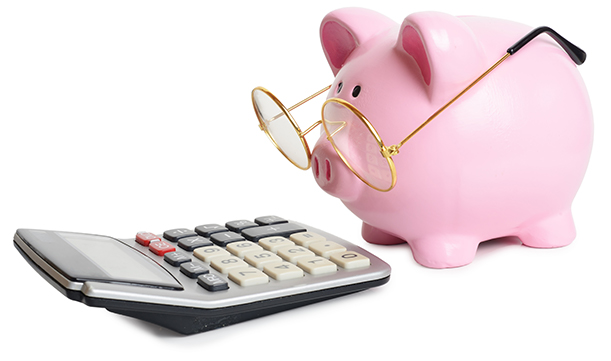
According to Gartner (January 2020), "By 2024, almost all traditional applications migrated to the Cloud as a Service (infrastructure as a service/IaaS) will require cost optimization to be truly efficient and profitable"
For the model to work, costs, and therefore uses, must be controlled.
And this is where the intelligently designed delegation of transformation can provide its share of solutions.
Controlling Cloud costs is based on two factors: taming and regulating uses.
Cloud data technologies each have their own billing method.
Controlling usage will consist in using each feature wisely and in the right way, so as to reduce usage to its strict minimum, and above all to prevent it getting out of hand. In other words, do as much, but using less or better, and without surprises.
Regulating use will consist of freeing resources based on actual use. Just at the right time, no more. The "serverless" architecture of the Cloud lends itself to this type of regulation, but the data integration solution must still be able to respect this type of architecture, which is far from easy when using an engine that runs continuously.
It is clear here that the delegation of transformation approach is an interesting alternative.
Like an orchestra conductor, it only plays each instrument at the necessary time, and regulates the nuances and harmonies, cadences the rhythm and the moments of silence.
Natively serverless, it has no reason to exist except for the need to optimize the use of resources. Its challenge now: the industrialization of optimizations.
Big Data, from craftsmanship to industrialization
Industrialization… concept which has been badly carried out lately.
But it was actually a necessity.
Big data, machine learning, AI, started with exploration phases, not to say taming.
You had to be of an adventurous nature to understand and take advantage of these technologies.
Its beginnings were marked by discovery, test phases and trials.
And, as in any innovation process, industrialization simply had no place yet.
Starting in "home-grown" mode turned out to be very appropriate and paid off.
The instruments had to be tuned.
These technologies have gradually found their place in the landscape of CIOs. Even if some are still in the experimental phase, others have tamed them, and others have moved on…
For those who have now integrated them, it is time to move on to the industrial phase, to move from concept to production.
And this is where the data integration tool must play its part.
The challenge is no longer the engine but the industrialization of transformation flows and algorithms.
When the score is written, it must be spread, democratized, and for data artists, put into music and practised.
Delegation of transformation is the preferred operating mode for these platforms.
However, this does not come down, as some seem to think, to simply knowing how to make technologies work.
The real ELT automates tasks and increases productivity, quality and reliability.
The good ELT of the 2020s is the ELT that industrializes, that saves time.
And time, we all need more of it! More time to draw new uses around the data.
If data transformation was crucial in the BI world of the 90s, it is even more so in the world of the data lake!
This is what we will explore in our next article: the role of transformation in data management systems..
Continuation of the article soon "Transformation, keystone of data management systems"
About the Author :
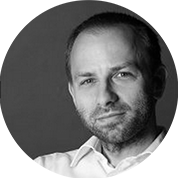
Fabien BRUDER, Co-founder of Stambia company since 2009.
EPITA computer engineer, specialized in artificial intelligence, graduated in business management from IAE Paris.
After IBS France (integrator) then Sagent (publisher), he spent 7 years with the publisher Sunopsis (Oracle), as a consultant, technical director and then agency director.
He has been working as an expert in the field of data integration for 20 years now.
Data integration solutions have gone from the man-orchestra to the conductor model.
But to play which score?
That of a well-tuned quartet? Or that of a chamber orchestra, playing a limited selection of instruments?